In the age of artificial intelligence, explainable AI is becoming a crucial component for unlocking valuable insights while ensuring transparency and accountability. You may think of AI systems as enigmatic “black boxes,” but with explainable AI, you gain a window into how these systems make decisions, shedding light on the intricacies of algorithmic processes. This increasing transparency in algorithms empowers you to understand the underpinnings of machine learning models, paving the way toward fairness and ethical AI practices.
Explainability techniques enhance your trust in AI, allowing you to discern biases and ensure data transparency. By leveraging transparency tools and frameworks, you play a vital role in fostering human-AI collaboration, aiding in the governance and regulation of AI models. Through the augmentation of model interpretability, you can delve deeper into AI decision-making processes, promoting responsible AI practices and equitable outcomes. Allow this deep learning transparency to be your guide in navigating the complexities of algorithmic transparency and accountability, ultimately contributing to a more transparent AI ecosystem.
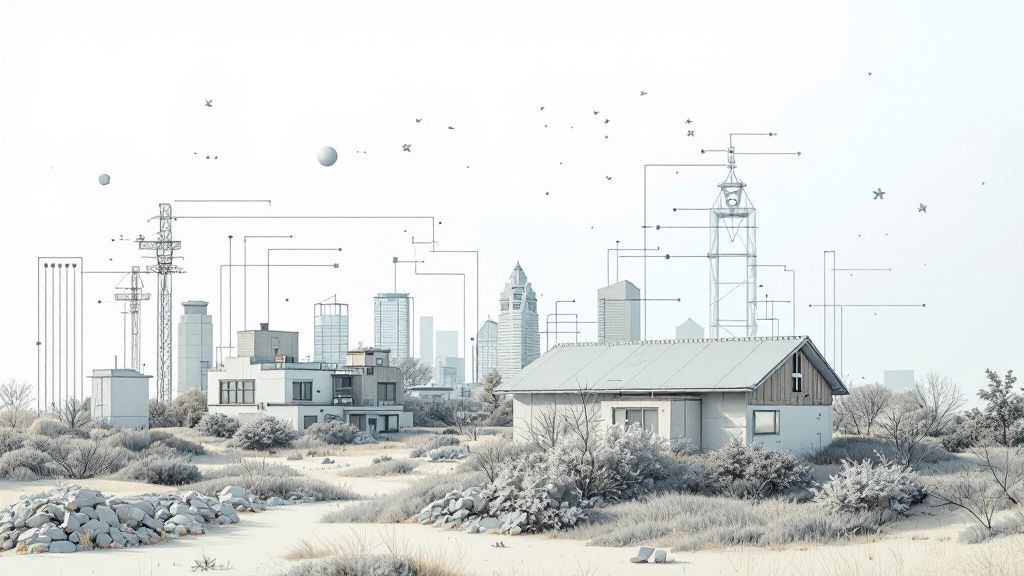
Understanding Explainability in the Context of AI
In the realm of artificial intelligence, explainability serves as the cornerstone for understanding and utilizing AI systems effectively. It bridges the gap between complex algorithms and human comprehension, allowing you to interpret how AI models generate outcomes. This understanding fosters algorithmic transparency and accountability, crucial elements in ensuring fair and ethical AI practices. By shedding light on data transparency and potential bias in AI, explainability enhances trust in AI decision-making, crucial for effective human-AI collaboration.
Insights from AI ethics expert Tim Miller suggest that interpretable machine learning is pivotal for advancing transparent AI systems. His views emphasize the importance of model interpretability in promoting fairness and tackling challenges related to deep learning transparency. Transparency tools, AI policy, and transparency frameworks are essential in achieving computational transparency. By embracing these elements, you can confidently engage with AI, promoting responsible AI governance and shaping the future landscape of AI technology.

The Rise of AI and the Need for Explanation
As AI technology continues to advance, the demand for explainable AI grows significantly. This demand acquires practical importance as you seek to unravel the seemingly mysterious workings of AI systems. The rise of AI technology prompts us to consider important questions about transparency in algorithms and machine learning transparency. Without clear understanding, AI's potential benefits might be overshadowed by concerns surrounding AI ethics and accountability.
How does the rise of artificial intelligence impact the trust of users in AI systems? This phenomenon is significant because trust in AI decision-making hinges on your ability to comprehend and evaluate the systems' processes. Algorithmic accountability and interpretable machine learning are essential for building this trust, ensuring that AI operates with fairness and ethical considerations at its core.
Explainability techniques play a pivotal role in bringing clarity to AI processes, reducing bias in AI and enhancing data transparency. By adopting these techniques, you can effectively manage risks associated with black-box AI and enable transparent AI systems. The rise of AI underscores the need for deeper engagement with transparency tools and frameworks to support ethical AI practices.
In the context of AI governance, establishing AI policy and regulation becomes crucial. This ensures that AI accountability and algorithmic fairness are maintained. The focus is shifting toward setting standards for computational transparency and model interpretability, helping you navigate the complexities of AI application with confidence.
As human-AI collaboration increases, the necessity for explainable deep learning and transparency in algorithms becomes apparent. Addressing these needs allows you to harness AI systems responsibly, aligning their growth with societal values and promoting an environment where ethical AI thrives.
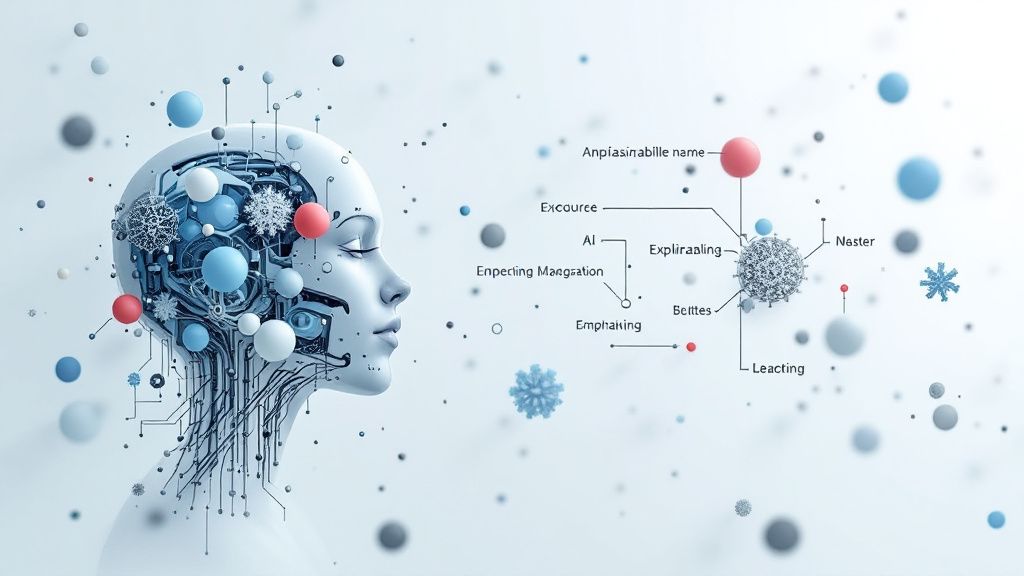
Core Principles of Explainable AI
Explainable AI is anchored on several core principles that guide its development and application, ensuring that you understand AI's decision-making processes. Transparency in algorithms is paramount, allowing you to see through the complexities of machine learning models. By fostering algorithmic transparency, you can minimize bias in AI systems, bolstering fairness in AI and reinforcing ethical AI frameworks.
To truly understand explainable AI, you need to shift your mindset from accepting opaque AI systems to demanding clarity and interpretability. Embracing this new perspective encourages the adoption of transparency tools and interpretable machine learning, which enhance your trust in AI decisions. This mindset shift paves the way for AI accountability and the responsible use of AI technologies.
Model interpretability and data transparency are essential facets of explainable AI. They provide you with insights into the factors influencing AI decisions, supporting your efforts to maintain algorithmic fairness. By focusing on deep learning transparency and computational transparency, you strengthen human-AI collaboration, creating transparent AI systems that align with societal values.
AI governance plays a crucial role in upholding the core principles of explainable AI. Establishing AI policy and regulation ensures that AI operates within ethical boundaries, safeguarding the integrity of AI decision-making processes. This upholds responsible AI practices, making AI technology a trustworthy ally in addressing complex challenges.

Why Explainability Matters in AI Technology
Explainability in AI technology bears immense significance as it directly impacts your ability to trust and effectively use AI systems. By enhancing transparency in algorithms, explainable AI demystifies AI decision-making, providing clarity and fostering a deeper understanding. This is crucial for ensuring fairness in AI, as it helps you identify and rectify any bias inherent in AI models, promoting ethical AI practices and algorithmic accountability.
The current state of AI technology shows a growing trend towards prioritizing machine learning transparency and explainability techniques. As you navigate this landscape, interpretable machine learning and model interpretability become invaluable, enabling you to engage responsibly with AI systems. Data transparency and transparency tools are increasingly being adopted, driven by an understanding that they are key to building trust in AI and enhancing human-AI collaboration.
AI governance reflects this shift, with more emphasis on AI accountability and regulation. The demand for transparent AI systems has inspired the creation of new policies and transparency frameworks, shaping a future where responsible AI use is the norm. As explainability grows in prominence, AI technology evolves to meet the ethical standards and expectations essential for advancing AI development while safeguarding societal values.
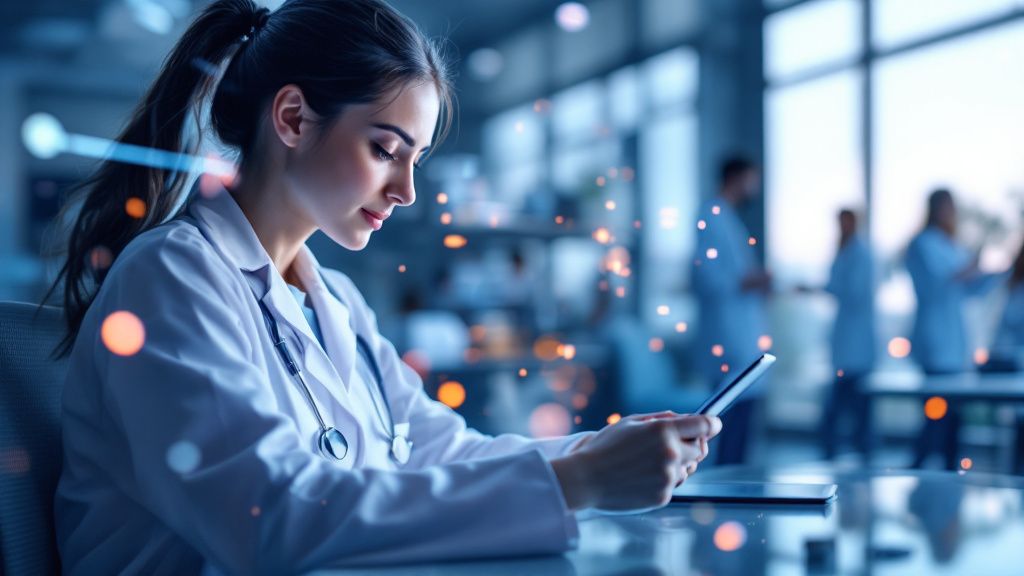
AI in Healthcare: The Role of Explainable Algorithms
AI in healthcare is transforming the way you experience medical services, where explainable algorithms play a pivotal role. They provide the clarity needed to understand complex AI-driven decisions, fostering trust in AI systems. By prioritizing transparency in algorithms and interpretable machine learning, healthcare can tackle challenges associated with data transparency and bias, ensuring ethical AI practices permeate throughout the industry.
What most people don’t see about AI in healthcare is the intricate process of explainability techniques that make all the difference. These techniques ensure that AI decision-making aligns with fairness in AI, promoting algorithmic accountability. The precision of model interpretability enhances your confidence in AI diagnostics, empowering human-AI collaboration to reach new heights.
Furthermore, explainable AI systems in healthcare support AI accountability and responsible use of AI technologies. The application of transparency tools bolsters computational transparency, paving the way for transparent AI systems that uphold patient care quality and safety standards. By embracing these practices, healthcare professionals can rely on AI technology to achieve more accurate and efficient outcomes.
As AI governance advances, the integration of AI policy and regulation in healthcare becomes crucial. Balancing algorithmic transparency with deep learning transparency is essential for strengthening AI applications in medical fields. By focusing on these elements, explainable algorithms can effectively revolutionize healthcare decision-making, supporting ethical AI use and improving patient satisfaction.

Finance Sector: Building Trust with AI Transparency
In the finance sector, the growing integration of artificial intelligence necessitates a strong emphasis on building trust through AI transparency. As you navigate through complex financial systems, explainable AI can enhance your understanding and trust in AI processes. Ensuring transparency in algorithms allows for effective AI decision-making and fosters algorithmic accountability in transactions and financial models.
Did you know? A staggering 60% of financial institutions acknowledge increased reliance on AI for decision-making, highlighting the need for machine learning transparency. By implementing transparency tools and interpretable machine learning, you can mitigate potential bias in AI systems, promoting fairness in AI and reinforcing ethical AI practices in financial operations.
Model interpretability and data transparency are key to fostering trust in AI within the finance sector. Explainability techniques illuminate the underlying processes, ensuring transparent AI systems remain accountable. By enhancing deep learning transparency, financial institutions enable human-AI collaboration, providing you with reliable, data-driven financial solutions.
AI governance in the finance sector is evolving to include robust AI policy and regulation. This focus on algorithmic fairness and explainable deep learning ensures that AI accountability is maintained. By championing transparency frameworks and computational transparency, the finance industry can harness responsible AI, fostering confidence and innovation in an evolving digital landscape.